Participatory multi-objective optimization for planning dense and green cities
In a recently published paper in the Journal of Environmental Planning and Management, we investigated the usage of a participatory multi-objective optimization to plan new residential areas to accommodate urban dwellers. These planning procedures increasingly require the consideration of urban ecosystem services (UES), especially when taking into account the irreversibility of urban development.
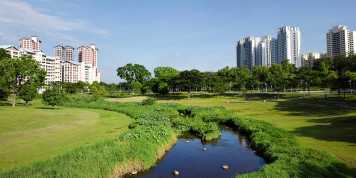
In a compact and land-scarce city like Singapore, the allocation of new residential areas poses a major challenge of high complexity. Designed to operationalize and solve such complex problems, optimization procedures can identify trade-offs between multiple objectives by displaying the optimal solutions in a so-called trade-off curve. A solution is considered optimal if an objective cannot be improved, without reducing another objective. Furthermore, multi-objective optimizations allow the integration of various perspectives of stakeholders into the modelling process, making them well-suited to be integrated in participatory approaches.
The multi-objective optimization approach applied in the frame of Natural Capital Singapore was designed to support decision-makers in their efforts to allocate new residential areas that minimize the loss of UES and maximize compactness. The resulting maps of the optimization show the pixels that are best suited to optimize both objectives simultaneously. Stakeholders can prioritise one objective over another by clicking on the different solutions on the generated trade-off curve. The participatory optimization approach aims to support negotiations about sustainable development options and about conflicting demands for the remaining green areas in Singapore. In a second step, we were able to show that the optimization framework can be extended to create temporal urban growth simulations linked to future transportation nodes. Future scenarios of urban growth patterns with a minimal loss of UES and maximized compactness of the urban fabric were generated.
We embedded our optimization approach in an interactive decision support tool to facilitate the collaborative and iterative solution-finding process. This approach not only fosters the acquisition of interdisciplinary knowledge but allows trade-offs between UES and compactness to be addressed explicitly, which is pivotal for shaping the growing cities across the world.
Read more here: external page https://doi.org/10.1080/09640568.2021.1875999