New publication about deep learning landscape typologies
The identification and definition of landscape types is not only essential for the transferability of landscape planning and management strategies, but also a requirement of the European Landscape Convention ratified by Switzerland. Landscape typologies are usually made by experts or with the use of so-called unsupervised clustering techniques.
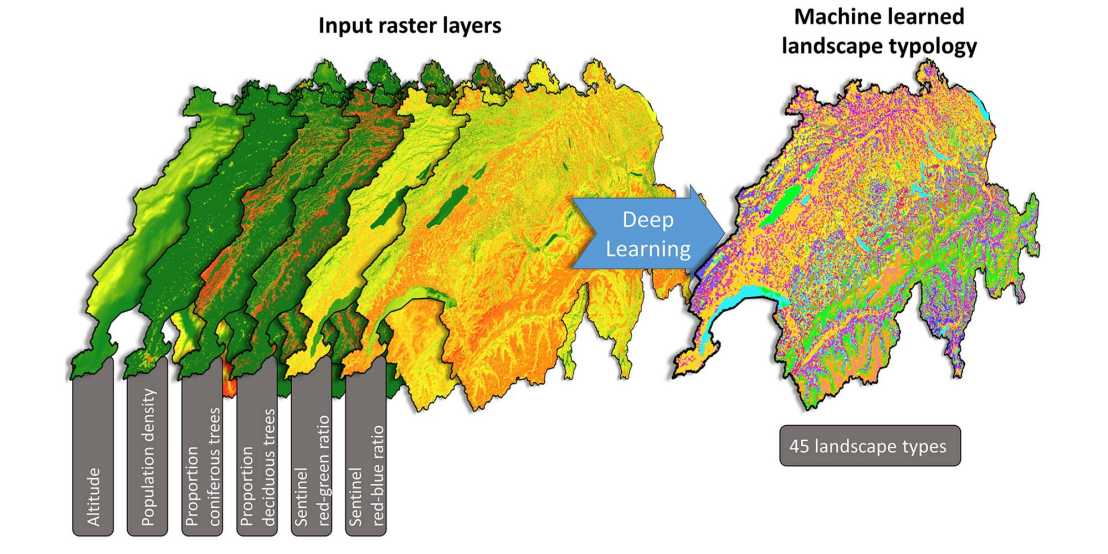
With the current clustering techniques, landscape patterns (i.e. an important landscape characteristic) are calculated from classified land-use/land-cover maps with landscape metrics. However, classified land-use/land-cover maps can be biased and are not useful to detect some of the fine-scaled landscape patterns that characterise a landscape. Making use of remote sensing satellite or aerial images and other continuous layers (e.g. digital elevation models) would enable the identification of such patterns. However, the landscape metrics usually used to quantify landscape patterns cannot be applied to such continuous datasets and quantify only a very narrow selection of potential patterns.
At PLUS, we are therefore experimenting with new machine learning techniques to classify landscapes based on patterns identified in continuous spatial data, such as satellite images and digital elevation models. For this, we do not make use of pre-existing landscape metrics, but we let computer algorithms search for patterns that distinguish landscapes from one another: a process that is called deep learning. In this new publication, we present a deep learning approach that can be used to automatically detect landscape types in continuous spatial data.
The article can be found on external page https://www.sciencedirect.com/science/article/pii/S1364815222001670